This topic contains additional examples of how to use more features of LabKey SQL:
Also see:
Query Columns with Duplicate Names
When joining two tables that have some column names in common, the duplicates will be disambiguated by appending "_1", "_2" to the joined column names as needed. The first time the column name is seen in the results, no number is appended.
For example, the results for this query would include columns named "EntityId", etc. from c1, and "EntityId_1", etc. from c2:
SELECT * FROM core.Containers c1 INNER JOIN core.Containers c2 ON
c1.parent = c2.entityid
Note that the numbers are appended to the
field key, not the
caption of a column. The user interface displays the caption, and thus may omit the underscore and number. If you hover over the column, you will see the field key, and if you export data with
"Column headers=Field key" the numbered column names will be included.
If you want to be able to display to users which field came from which table, you can edit the originating table definition to add either a distinct field label, or a
Description including the origin of the field, which will be shown to users on hover.
"Datasets" Special Column in Studies
SQL queries based on study datasets can make use of the special column "Datasets", which gives access to all datasets in the current study.
For example, the following query on the PhysicalExam dataset uses the "Datasets" special column to pull in data from the Demographics dataset. You can use this query in the
tutorial example study.
SELECT PhysicalExam.ParticipantId,
PhysicalExam.Weight_kg,
PhysicalExam.Datasets.Demographics.Height,
PhysicalExam.Datasets.Demographics.Country
FROM PhysicalExam
"Datasets" provides a shortcut for queries that would otherwise use a JOIN to pull in data from another dataset. The query above can be used instead of the JOIN style query below:
SELECT PhysicalExam.ParticipantId,
PhysicalExam.Weight_kg,
Demographics.Height,
Demographics.Country
FROM PhysicalExam
JOIN Demographics
ON PhysicalExam.ParticipantId = Demographics.ParticipantId
Use "GROUP BY" for Calculations
The GROUP BY function is useful when you wish to perform a calculation on a table that contains many types of items, but keep the calculations separate for each type of item. You can use GROUP BY to perform an average such that only rows that are marked as the same type are grouped together for the average.
For example, what if you wish to determine an average for each participant in a large study dataset that spans many participants and many visits. Simply averaging a column of interest across the entire dataset would produce a mean for all participants at once, not each participant. Using GROUP BY allows you to determine a mean for each participant individually.
GROUP BY Example: Average Temp Per Participant
The GROUP BY function can be used on the PhysicalExam dataset to determine the average temperature for each participant across all of his/her visits.
To set up this query, follow the basic steps described in the
Create a SQL Query example to create a new query based on the PhysicalExam table in the study schema. Name this new query "AverageTempPerParticipant."
If you are working with the LabKey tutorial study, these queries may be predefined, so you can view and edit them in place, or create new queries with different names.
Within the SQL Source editor, delete the SQL created there by default for this query and paste in the following SQL:
SELECT PhysicalExam.ParticipantID,
ROUND(AVG(PhysicalExam.temperature_c), 1) AS AverageTemp,
FROM PhysicalExam
GROUP BY PhysicalExam.ParticipantID
For each ParticipantID, this query finds all rows for that ParticipantID and calculates the average temperature for these rows, rounded up to the 10ths digit. In other words, we calculate the participant's average temperature across all visits and store that value in a new column called "AverageTemp."
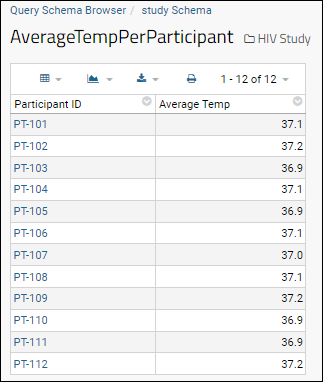
See also the
Efficient Use of "GROUP BY" section below.
JOIN a Calculated Column to Another Query
The JOIN function can be used to combine data in multiple queries. In our example, we can use JOIN to append our newly-calculated, per-participant averages to the PhysicalExam dataset and create a new, combined query.
First, create a new query based on the "PhysicalExam" table in the study schema. Call this query "PhysicalExam + AverageTemp" and edit the SQL to look like:
SELECT PhysicalExam.ParticipantId,
PhysicalExam.date,
PhysicalExam.weight_kg,
PhysicalExam.temperature_C,
PhysicalExam.systolicBP,
PhysicalExam.diastolicBP,
PhysicalExam.pulse,
AverageTempPerParticipant.AverageTemp
FROM PhysicalExam
INNER JOIN AverageTempPerParticipant
ON PhysicalExam.ParticipantID=AverageTempPerParticipant.ParticipantID
You have added one line before the FROM clause to add the AverageTemp column from the AverageTempPerParticipant dataset. You have also added one additional line after the FROM clause to explain how data in the AverageTempPerParticipant are mapped to columns in the PhysicalExam table. The ParticipantID column is used for mapping between the tables.
Premium Resource AvailableSubscribers to premium editions of LabKey Server can learn about displaying a calculated column in the original table following the example in this topic:
Learn more about premium editions
Calculate a Column Using Other Calculated Columns
We next use our calculated columns as the basis for creating yet another calculated column that provides greater insight into our dataset.
This column will be the difference between a participant's temperature at a particular visit and the average temperature for all of his/her visits. This "TempDelta" statistic will let us look at deviations from the mean and identify outlier visits for further investigation.
Steps:
- Create a new query named "PhysicalExam + TempDelta" and base it on the "PhysicalExam + AverageTemp" query we just created above.
- Paste the following SQL:
SELECT "PhysicalExam + AverageTemp".ParticipantId,
"PhysicalExam + AverageTemp".date,
"PhysicalExam + AverageTemp".weight_kg,
"PhysicalExam + AverageTemp".temperature_c,
"PhysicalExam + AverageTemp".systolicBP,
"PhysicalExam + AverageTemp".diastolicBP,
"PhysicalExam + AverageTemp".pulse,
"PhysicalExam + AverageTemp".AverageTemp,
ROUND(("PhysicalExam + AverageTemp".temperature_c-
"PhysicalExam + AverageTemp".AverageTemp), 1) AS TempDelta
FROM "PhysicalExam + AverageTemp"
- Provide a longer display name for the new column by pasting this content on the XML Metadata tab.
<tables xmlns="http://labkey.org/data/xml">
<table tableName="PhysicalExam + TempDelta" tableDbType="NOT_IN_DB">
<columns>
<column columnName="TempDelta">
<columnTitle>Temperature Difference From Average</columnTitle>
</column>
</columns>
</table>
</tables>
Filter Calculated Column to Make Outliers Stand Out
It can be handy to filter your results such that outlying values stand out. This is simple to do in a LabKey grid using the
column header filtering options.
Using the query above ("PhysicalExam + TempDelta"), we want to show the visits in which a participant's temperature was unusually high for them, possibly indicating a fever. We filter the calculated "Temperature Difference From Average" column for all values greater than 1.5. Just click on the column header, select
Filter. Choose "Is Greater Than" and type "1.0" in the popup, then click
OK.
This leaves us with a list of all visits where a participant's temperature was more than 1 degree C above the participant's mean temperature at all his/her visits. Notice the total number of filtered records is displayed above the grid.
Use "SIMILAR_TO"
String pattern matching can be done using similar_to. The syntax is similar_to(A,B,C): A similar to B escape C. The escape clause is optional.
- 'A' is the string (or field name) to compare
- 'B' is the pattern to match against
- 'C' is the escape character (typically a backslash) used before characters that would otherwise be parsed as statement syntax, including but not limited to "%", "(", ",".
To return all the names on a list that started with AB, you might use:
SELECT Name from MyList
WHERE similar_to (Name, 'AB%')
If you wanted to return names on the list that started with '%B', you would use the following which uses a to escape the first % in the string.:
WHERE similar_to (Name, '\%B%', '\')
Learn more about SQL matching functions
here.
Match Unknown Number of Spaces
If you have data where there might be an arbitrary number of spaces between terms that you want to match in a pattern string, use SIMILAR_TO with a space followed by + to match an unknown number of spaces. For example, suppose you wanted to find entries in a list like this, but didn't know how many spaces were between the term and the open parentheses "(".
- Thing (one)
- Thing (two - with two spaces)
- Thing to ignore
- Thing (three)
Your pattern would need to both include " +" to match one or more spaces and escape the "(":
WHERE similar_to(value, 'Thing +\(%', '\')
Round a Number and Cast to Text
If your query involves both rounding a numeric value (such as to 1 or two digits following the decimal place) and also casting that value to text, the CAST to VARCHAR will "undo" the rounding adding extra digits to your text value. Instead, use
to_char. Where 'myNumber' is a numeric value, pass the formatting to use as the second parameter. to_char will also round the value to the specified number of digits:
to_char(myNumber,'99999D0')
The above format example means up to 5 digits (no leading zeros included), a decimal point, then one digit (zero or other).
More formats for numeric values are available here.
Efficient Use of "GROUP BY"
In some use cases, it can seem logical to have a long GROUP BY list. This can be problematic when the tables are large, and there may be a better way to write such queries both for performance and for readability. As a general rule, keeping GROUP BY clauses short is best practice for more efficient queries.
As an example, imagine you have customer and order tables. You want to get all of the customer's info plus the date for their most recent order.
You could write something like:
SELECT c.FirstName, c.LastName, c.Address1, c.Address2, c.City, c.State, c.Zip, MAX(o.Date) AS MostRecentOrder
FROM
Customer c LEFT JOIN Order o ON c.Id = o.CustomerId
GROUP BY c.FirstName, c.LastName, c.Address1, c.Address2, c.City, c.State, c.Zip
...but that makes the database do the whole join (which may multiply the total number of rows) and then sort it by all of the individual columns so that it can de-duplicate them again for the GROUP BY.
Instead, you could get the most recent order date via a correlated subquery, like:
SELECT c.FirstName, c.LastName, c.Address1, c.Address2, c.City, c.State, c.Zip, (SELECT MAX(o.Date) FROM Order o WHERE c.Id = o.CustomerId) AS MostRecentOrder
FROM
Customer c
Or, if you want more than one value from the Order table, change the JOIN so that it does the aggregate work:
SELECT c.FirstName, c.LastName, c.Address1, c.Address2, c.City, c.State, c.Zip, o.MostRecentOrder, o.FirstOrder
FROM
Customer c LEFT JOIN (SELECT CustomerId, MAX(Date) AS MostRecentOrder), MIN(Date) AS FirstOrder FROM Order GROUP BY CustomerId) o ON c.Id = o.CustomerId
"COALESCE" Same-named Columns
If you have multiple data structures that are similar in having some of the "same" fields, but stored in distinct structures, you cannot directly join these fields in a query, but provided they are of the same type, you can use COALESCE to 'fold' them together.
For example, say you have two Sample Types, "Blood" and "Plasma" and each has a "MAIN_ID" field and a "Date" field. You want to print a grid to be used to make an inventory list for a mixed box of samples. You can add samples of both types to a list (keyed on the "Name" field of each sample), such as a Picklist in Sample Manager or Biologics. Any multi-Sample Type grid will include fields common to all Sample Types on one tab, but this is limited to built in fields that the system has internally aligned. In this example, we're adding additional fields but the system does not have a way of knowing that they are "related".
You could print a multi-sheet Excel file from a picklist, separating the fields for the two types from within the application. In order to create a "combined" grid showing the corresponding values of these Sample Type-specific fields in one place, you could use "COALESCE" to combine the different table columns into one:
SELECT PrintThisBox.SampleID,
PrintThisBox.SampleId.SampleSet.Name As "Sample Type",
COALESCE(Blood.MAIN_ID, Plasma.MAIN_ID, 'None') AS MAIN_ID,
COALESCE(Blood.Date, Plasma.Date) AS "Sample Date"
FROM PrintThisBox
LEFT JOIN samples.Blood on PrintThisBox.SampleId.Name = Blood.Name
LEFT JOIN samples.Plasma on PrintThisBox.SampleId.Name = Plasma.Name
In this example, we're making use of a picklist named "PrintThisBox" containing samples of the two types. The "MAIN_ID" field in each Sample Type will be COALESCED into a "blended" MAIN_ID column, with any rows failing to have values for it shown as 'None'. In our example, we know that the "Date" field is always populated, so don't need to provide a default value. As many tables as needed could be "folded" into a shared set of columns in this manner, as COALESCE will look in each row for the first one of the provided arguments that exists.
Related Topics